The Ultimate Guide to Image Classification Labeling Tools
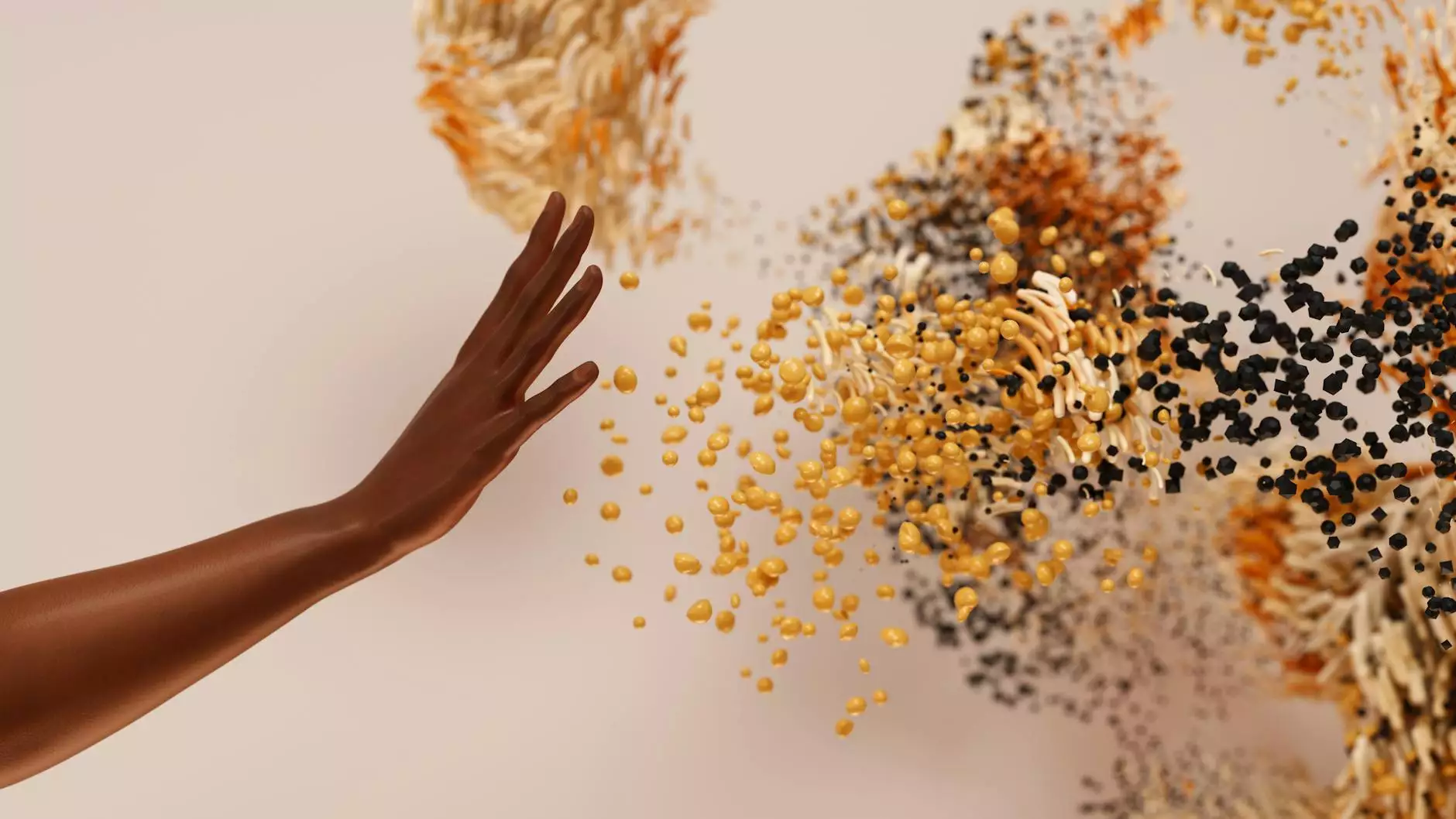
In today's rapidly evolving technological landscape, the demand for high-quality data annotation continues to rise, particularly in sectors driven by artificial intelligence and machine learning. Among the pivotal components of this domain is the image classification labeling tool, which plays a significant role in training models to recognize and categorize images accurately.
Understanding Image Classification Labeling Tools
Image classification is a vital process in machine learning that involves identifying the content of an image and assigning it predefined labels. The image classification labeling tool serves as a bridge between raw image data and the machine learning models that use this data to learn and make predictions.
These tools enable users to annotate images effectively, ensuring that the resulting datasets are well-organized, precise, and readily applicable for training models. With the right tool, businesses can enhance their operational efficiency, reduce the time needed for data preparation, and improve the overall quality of their machine learning outputs.
The Importance of Data Annotation in AI Development
As companies strive to implement artificial intelligence in their processes, the need for robust data annotation becomes critical. Here are several reasons illustrating the importance of data annotation:
- Quality Model Training: Machine learning models learn from labeled data. The more accurate the labeling, the better the model performs.
- Scalability: As businesses grow, the volume of data increases exponentially. Efficient labeling tools help manage this data influx without compromising quality.
- Enhanced Accuracy: Automated labeling tools often incorporate AI to assist human annotators, enhancing labeling accuracy and consistency.
- Versatility: Image classification labeling tools are applicable across various sectors, including healthcare, automotive, retail, and more, allowing businesses to adapt to different needs.
Key Features of Effective Image Classification Labeling Tools
When selecting an image classification labeling tool, certain features are essential to ensure you are investing in a product that meets your needs. Here are some key features to consider:
1. User-Friendly Interface
A well-designed interface allows annotators to work more efficiently. Look for tools that provide an intuitive layout, enabling users to annotate images quickly and accurately.
2. Support for Various Data Types
Modern annotation tools should support multiple image formats, including JPG, PNG, and TIFF. Additionally, support for video and 3D data can provide added flexibility.
3. Collaboration Features
To ensure team members can work together efficiently, select a tool that offers collaboration features, allowing multiple users to annotate and review images simultaneously.
4. Quality Control Mechanisms
Implementing quality control measures is crucial. Look for tools that offer validation features, allowing users to review and verify annotations to maintain high-quality standards.
5. Integration Capabilities
The ability to integrate with other software solutions, such as machine learning frameworks or project management tools, can streamline workflows and improve efficiency.
Getting Started with Image Classification Labeling Tools
To leverage the benefits of image classification labeling tools, businesses should follow these steps:
Step 1: Define Your Objectives
Before choosing a tool,明确项目目标,以确保所选软件适合特定需求。例如,您可能需要注释用于物体检测、面部识别或场景分类的数据。
Step 2: Select the Right Tool
根据所识别的目标和上面强调的特征,评估不同的工具。有许多开源和商业选项,确保选择最符合需求的解决方案。
Step 3: Train Your Team
确保Annotation团队了解所选工具的功能和工作流程。提供培训和资源,以帮助他们有效使用工具。
Step 4: Start Annotating
一旦团队准备就绪,开始处理数据。遵循预先定义的标准,以保持注释质量的一致性。
Step 5: Review and Verify
实施审查流程,以确保所有标记都是准确的。如果可能,使用自动化工具来辅助验证注释。
Integrating Image Classification Labeling Tools into Your Business Operations
将图像分类标记工具集成到您的业务运营中将极大地增强您的工作流程。以下是实现集成的几个策略:
- 与数据管道的集成:确保标记工具可以直接与您的数据源连接。此步骤将消除手动数据传输的需要。
- 采用API接口:寻找支持API集成的工具,以更轻松地将标记过程纳入您的技术堆栈。
- 自动化数据存储:标记后的数据应自动存储在一致的位置,便于以后的检索和使用。
- 培训与支持:定期培训团队以熟悉新工具能够极大提高效率,并确保每个人都在最新状态。
Challenges in Image Classification Labeling
尽管有许多好处,但图像分类标记工具也面临一些挑战,包括:
1. Managing Large Volumes of Data
随着数据量的持续增加,标记过程很容易变得繁琐。选择支持批量处理和自动化功能的工具可以帮助解决这个问题。
2. Ensuring Annotation Consistency
不同的注释者可能会产生不同的结果。实现一致的标记标准并使用质量控制措施对于确保数据集的一致性至关重要。
3. Time Constraints
在紧迫的截止日期下,保持高质量注释可能是一项挑战。有效的项目管理和分配资源是解决此问题的关键。
Future Perspectives of Image Classification Labeling Tools
随着人工智能行业的不断发展,图像分类标记工具也在不断进化。以下是一些即将出现的趋势:
- 自动化提升:随着AI和机器学习的进步,许多工具将实现更高程度的自动化,减少对人工标记的依赖。
- 多模态数据支持:将来,标注工具将支持更广泛类型的数据,包括文本、音频和视频,允许跨部门的更全面分析。
- 用户定制选项:更多选择将赋予用户调整工具以符合其特定工作流的能力,创建更加个性化的体验。
- 提高隐私和合规性:随着对数据隐私的关注加剧,未来的工具将更加专注于合规性及安全操作。
Conclusion
总结一下,选择和实施一个图像分类标记工具对于任何希望在人工智能和机器学习领域取得成功的公司来说都是至关重要的。通过理解这些工具的功能和重要性,您可以优化数据处理过程,从而保证更高效的数据分析、模型开发和最终成果。
与希望进入这一领域的行业相比,keylabs.ai 的数据注释工具和平台确保了满足现代企业对高质量标记数据的需求。如果您准备好提升您的操作并启动您的AI项目,了解keylabs.ai 提供的解决方案将是您迈向成功的关键一步。