Optimizing Email Security: Spam Filtering Using Machine Learning
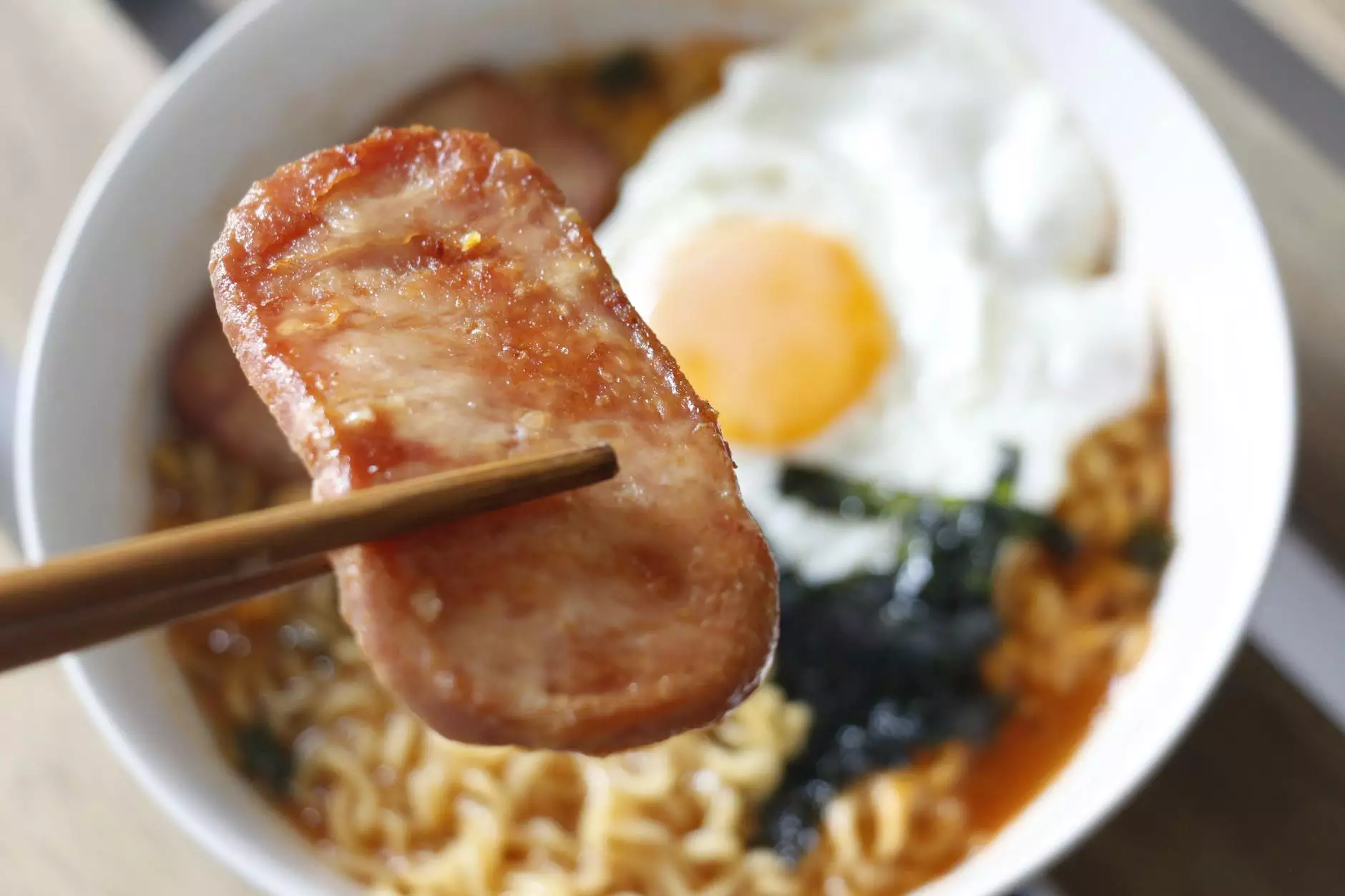
The Importance of Email Security in Modern Business
In today’s digital age, the significance of email security cannot be overstated. Businesses rely heavily on email communication, making them vulnerable to various threats, including spam, phishing, and malware attacks. The rise of sophisticated cyber threats necessitates robust security measures to protect sensitive information and maintain operational integrity.
Spam emails are not just an annoyance; they can lead to severe security breaches and data loss. Consequently, organizations must adopt advanced techniques for safeguarding their email systems. One of the most effective methods for achieving this is through spam filtering using machine learning.
Understanding Spam Filtering and its Evolution
Spam filtering is the process of identifying and blocking unwanted or harmful emails before they reach the recipient’s inbox. Traditional filtering methods relied heavily on predefined rules and keyword matching. However, spammers constantly evolve their tactics, often bypassing these basic filters.
To counteract this, modern spam filtering solutions have increasingly embraced machine learning, a branch of artificial intelligence that empowers systems to learn from data patterns and improve over time. This technology has revolutionized the way businesses manage email security.
How Machine Learning Enhances Spam Filtering
Machine learning algorithms analyze vast amounts of email data to identify characteristics common to spam messages. By doing so, they can effectively discern between legitimate emails and unwanted spam.
Key Techniques in Machine Learning for Spam Filtering
- Supervised Learning: This technique involves feeding the algorithm labeled datasets containing both spam and non-spam emails. The model learns from these examples and can classify new emails accurately.
- Unsupervised Learning: Unlike supervised learning, this approach does not use labeled data. The algorithm identifies patterns and clusters within the email data, which helps in detecting anomalies that may indicate spam.
- Neural Networks: Advanced methods such as deep learning utilize neural networks to process complex relationships within data. This enhances the model's ability to detect intricate patterns indicative of spam.
- Natural Language Processing (NLP): NLP techniques enable the algorithm to understand the context and semantics of email content, thereby improving its ability to filter out spam effectively.
Benefits of Using Machine Learning for Spam Filtering
Adopting spam filtering using machine learning offers numerous advantages for businesses, including:
- Improved Accuracy: Machine learning models continuously learn and adapt, resulting in higher accuracy in identifying spam compared to traditional methods.
- Real-time Filtering: These algorithms can analyze incoming emails in real-time, ensuring that harmful messages are blocked before reaching the inbox.
- Reduced False Positives: Advanced algorithms minimize the chances of legitimate emails being incorrectly categorized as spam, ensuring that important communications are not missed.
- Adaptive Learning: The system evolves with changing spam tactics, meaning it can recognize new types of threats without needing constant manual updates.
- Cost Efficiency: By reducing the risks associated with spam and phishing attacks, businesses save on potential losses from data breaches, spam-related downtime, and reputational damage.
Implementing Machine Learning Spam Filtering Solutions
Businesses looking to implement spam filtering using machine learning should follow a strategic approach:
1. Assess Email Security Needs
Conduct a thorough analysis of the current email security infrastructure to determine vulnerabilities and specific needs.
2. Choose the Right Machine Learning Model
Select an appropriate machine learning model based on the analysis – options include supervised, unsupervised, ensemble methods, or deep learning techniques.
3. Data Preparation and Training
Curate and label datasets for training the model. This step is crucial for supervised learning approaches, where quality labeled data significantly impacts model performance.
4. Continuous Monitoring and Updates
After deployment, continuously monitor the model’s performance. Regularly updating the model with new data helps maintain its accuracy and effectiveness over time.
5. Integration with Existing Systems
Ensure that the machine learning spam filtering solution integrates seamlessly with your existing email systems and enhances overall security without disrupting business operations.
Case Studies: Success Stories of Machine Learning Spam Filtering
Case Study 1: Fortune 500 Company
One Fortune 500 company implemented a machine learning-based spam filtering system and reported a 50% reduction in spam emails reaching employees' inboxes. The system learned from ongoing user feedback, constantly refining its accuracy.
Case Study 2: E-commerce Platform
An e-commerce platform utilized a hybrid machine learning model to filter spam and phishing attempts. This resulted in a 70% drop in reported phishing incidents and an increase in customer trust and engagement.
The Future of Spam Filtering: Trends to Watch
The future of email security lies in enhanced artificial intelligence capabilities. Here are some emerging trends in spam filtering using machine learning:
- Contextual Spam Filtering: Future algorithms will focus more on understanding the context of emails, leading to even better identification of malicious content.
- Behavioral Analysis: Monitoring how users interact with emails can provide insights into potential spam threats based on user behavior.
- Integration with Other Security Tools: Combining spam filtering with broader cybersecurity measures will create a more robust security environment.
Choosing Spambrella for Your Email Security Needs
At Spambrella, we specialize in providing comprehensive IT services and computer repair, alongside cutting-edge security systems tailored for today's challenges. Our machine learning-driven spam filtering solutions are designed to protect your organization from the evolving landscape of cyber threats.
We understand that each business is unique; thus, we focus on custom solutions that meet your specific needs. Our team of experts will work closely with you to ensure optimal setup, continuous monitoring, and updates to keep your email systems secure.
Contact us today to learn how Spambrella can enhance your email security with our proven spam filtering using machine learning technology.
© 2023 Spambrella. All rights reserved.