The Critical Role of Data Labeling in Machine Learning for Business Growth
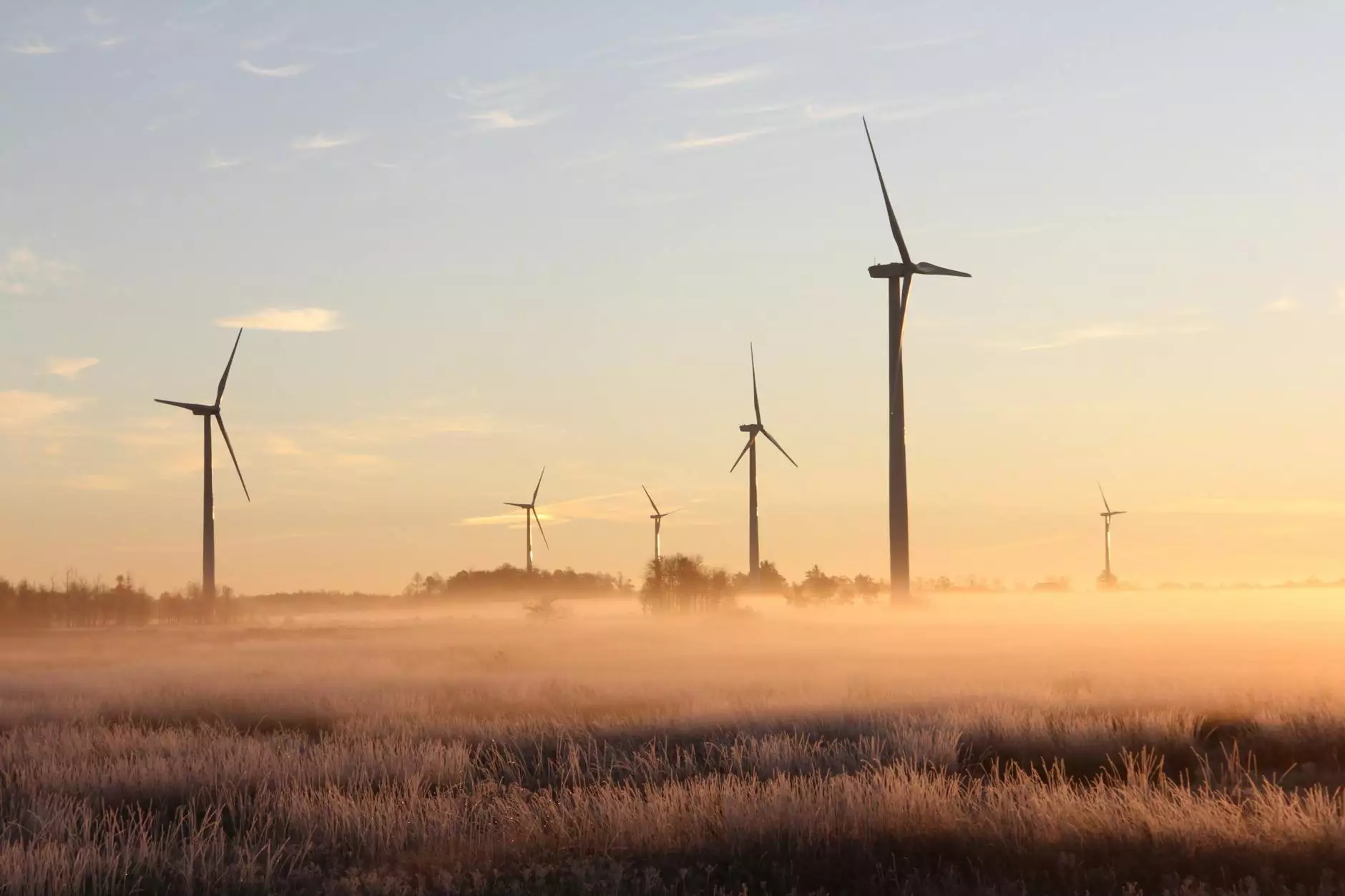
In the age of artificial intelligence and machine learning, data labeling has emerged as a foundational component for businesses looking to harness the full potential of these technologies. At KeyLabs.ai, we understand that precise and comprehensive data annotation is not just a technical procedure but a strategic business advantage. In this article, we will explore the significance of data labeling in machine learning, the tools and platforms available, and how they can seamlessly integrate into your business processes.
What is Data Labeling?
Data labeling involves the process of annotating data to create comprehensive datasets that machine learning models can learn from. In simpler terms, it's about ensuring that the algorithms understand the information they are trained on. This can include categorizing images, transcribing audio, tagging text, and much more. The better the quality of the labeled data, the more accurate the machine learning models will be.
Why is Data Labeling Essential for Machine Learning?
Data labeling serves multiple critical functions in the realm of machine learning:
- Improved Model Accuracy: High-quality labeled data directly correlates with improved accuracy in model predictions.
- Quality Assurance: Labeling helps in refining datasets, ensuring that the data fed into the machine learning model is representative and of high quality.
- Enables Supervised Learning: Supervised learning relies heavily on labeled datasets to train models effectively.
- Scalability: Efficient labeling processes allowing for quick adaptations and updates as data expands.
The Process of Data Labeling in Machine Learning
The process of data labeling can be broken down into several key steps:
- Data Collection: Gather the raw data from various sources. This could include images, videos, audio files, or textual information.
- Labeling Guidelines: Establish comprehensive guidelines that dictate how the data should be labeled. This helps ensure consistency across the dataset.
- Annotation: Use data labeling tools or platforms to annotate the data as per the established guidelines.
- Quality Control: Implement checks and balances to validate the labeling accuracy. Review labeled data for consistency and correctness.
- Training the Model: Use the labeled data to train machine learning models and continually refine the model's performance with new data.
Key Labs AI: Your Partner in Data Annotation Tool and Platform
At KeyLabs.ai, we provide cutting-edge data annotation tools designed to streamline the data labeling process. Our platform is built with state-of-the-art technology that supports:
- Customizable Workflow: Adapt our tools to suit the unique requirements of your project.
- Scalable Solutions: Handle large datasets efficiently with our automated labeling capabilities.
- Collaboration Features: Facilitate teamwork among annotators to improve throughput and consistency.
Types of Data Labeling Techniques
Understanding the different types of data labeling techniques can help businesses select the best approach for their needs. The most common techniques include:
- Image Annotation: Assigning labels to images for computer vision tasks. This includes bounding boxes, polygonal segmentation, and image classification.
- Text Annotation: Labeling textual data for natural language processing. Types include entity recognition, sentiment analysis, and text categorization.
- Audio Annotation: Transcribing and labeling audio files for speech recognition systems.
- Video Annotation: Labeling video content for activities can be used in various applications like surveillance, autonomous driving, and behavior analysis.
The Benefits of Using Data Annotation Platforms
Utilizing a dedicated data annotation platform offers numerous advantages:
- Efficiency: Automated tools can significantly reduce the time required for data labeling, allowing your team to focus on other critical tasks.
- Quality Assurance: Many platforms incorporate quality checks and ensure that data is consistently labeled, reducing errors and improving the overall quality of the dataset.
- Access to Expertise: Partnering with a specialized platform provides access to a team of expert annotators who understand the nuances of effective labeling.
- Cost-Effectiveness: While hiring in-house annotators can be expensive, outsourcing data labeling to a professional platform can often be more economical.
Challenges in Data Labeling and How to Overcome Them
Despite its importance, businesses can face several challenges in data labeling:
1. High Volume of Data
As businesses accumulate vast amounts of data, labeling it becomes a formidable task. To tackle this, companies can leverage automated labeling tools available in platforms like KeyLabs.ai.
2. Inconsistent Labeling
If different annotators label data differently, it can create significant issues. Establishing clear guidelines and conducting regular quality checks can mitigate this problem.
3. Domain Specificity
Certain datasets require a deep understanding of specific domains. Collaborating with domain experts during the labeling process can ensure accuracy and relevancy.
Best Practices for Effective Data Labeling
To maximize the effectiveness of your data labeling efforts, consider the following best practices:
- Define Clear Objectives: Establish the end goals of your machine learning project before starting the labeling process to guide decisions on labeling.
- Utilize Technology: Take advantage of tools that can automate various aspects of labeling to improve speed and accuracy.
- Ongoing Training: Provide ongoing training for annotators to keep them updated on the latest best practices and technologies.
- Feedback Loop: Implement a feedback mechanism to continually improve the labeling process based on outcomes and observations.
Conclusion: The Future of Data Labeling and Machine Learning
As businesses increasingly rely on machine learning to drive decision-making and efficiency, the importance of data labeling in machine learning cannot be understated. Investing in the right annotation tools and platforms, such as those provided by KeyLabs.ai, is crucial for businesses aiming to leverage artificial intelligence capabilities effectively.
In summary, the combination of sophisticated data labeling, robust machine learning models, and strategic business implementation stands to offer a competitive edge in today's data-driven marketplace. Emphasizing quality data annotation will not only enhance model performance but also pave the way for innovative solutions that can transform businesses across industries.
Take Action Today
To explore how KeyLabs.ai can assist your business with effective data labeling and provide you with top-notch data annotation tools, contact us today. Unlock the potential of your data and drive your machine learning initiatives forward!
data labeling machine learning